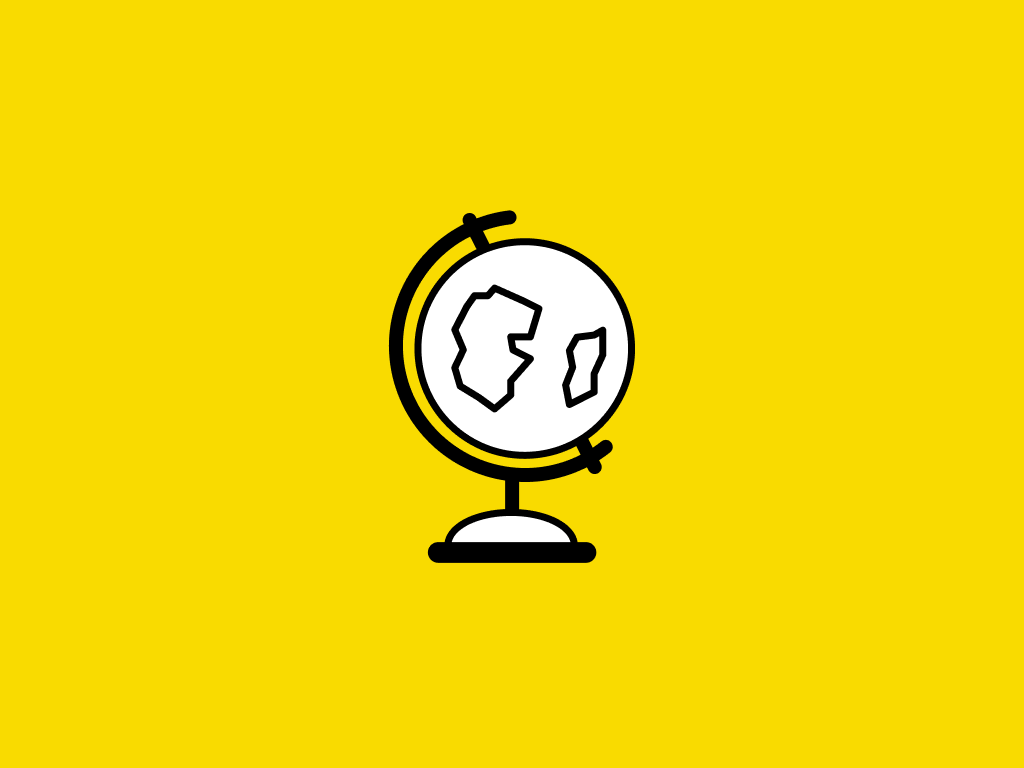
Description
A strong foundation in mathematics is critical for success in all science and engineering disciplines. Whether you want to make a strong start to a master’s degree, prepare for more advanced courses, solidify your knowledge in a professional context or simply brush up on fundamentals, this course will get you up to speed.
In many engineering master’s programs, statistics is used quite intensively. As soon as you are dealing with real-life data, you will need to get an idea of what these data tell you and how you can visualize this (descriptive statistics). But you will also want to perform some analysis (inferential statistics): you may want to build a model that mimics reality, estimate some quantities, or test some hypotheses.
The statistics course in this series will help you refresh your knowledge on these topics. Along the way you will learn how to apply these concepts to datasets, using the statistical software R.
This course offers enough depth to cover the statistics you need to succeed in your engineering master’s or profession in areas such as machine learning, data science and more.
This is a review course
This self-contained course is modular, so you do not need to follow the entire course if you wish to focus on a particular aspect. As a review course you are expected to have previously studied or be familiar with most of the material. Hence the pace will be higher than in an introductory course.
This format is ideal for refreshing your bachelor level mathematics and letting you practice as much as you want. You will get many exercises, to be solved using Grasple or R, for which you will receive intelligent, personal and immediate feedback.
Tags
Syllabus
Week 1: Descriptive statistics
- graphical summaries of datasets
- numerical summaries of datasets
- connection with probability theory
Week 2: Estimator theory
- quality of estimators
- methods to obtain estimators
Week 3: Hypothesis testing
- concepts
- how to perform a test in various settings
Week 4: Confidence intervals (CI)
- motivation
- how to construct a CI in various settings
Week 5: Linear regression
- simple and multiple linear regression
- categorical variables
- interpreting output
Week 6: Bootstrap and resampling
- parametric and non-parametric approach
- how to deal with non-standard situations
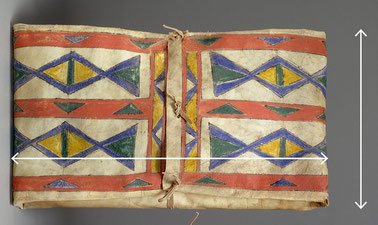
-
TypeOnline Courses
-
ProviderEdX
A strong foundation in mathematics is critical for success in all science and engineering disciplines. Whether you want to make a strong start to a master’s degree, prepare for more advanced courses, solidify your knowledge in a professional context or simply brush up on fundamentals, this course will get you up to speed.
In many engineering master’s programs, statistics is used quite intensively. As soon as you are dealing with real-life data, you will need to get an idea of what these data tell you and how you can visualize this (descriptive statistics). But you will also want to perform some analysis (inferential statistics): you may want to build a model that mimics reality, estimate some quantities, or test some hypotheses.
The statistics course in this series will help you refresh your knowledge on these topics. Along the way you will learn how to apply these concepts to datasets, using the statistical software R.
This course offers enough depth to cover the statistics you need to succeed in your engineering master’s or profession in areas such as machine learning, data science and more.
This is a review course
This self-contained course is modular, so you do not need to follow the entire course if you wish to focus on a particular aspect. As a review course you are expected to have previously studied or be familiar with most of the material. Hence the pace will be higher than in an introductory course.
This format is ideal for refreshing your bachelor level mathematics and letting you practice as much as you want. You will get many exercises, to be solved using Grasple or R, for which you will receive intelligent, personal and immediate feedback.
Week 1: Descriptive statistics
- graphical summaries of datasets
- numerical summaries of datasets
- connection with probability theory
Week 2: Estimator theory
- quality of estimators
- methods to obtain estimators
Week 3: Hypothesis testing
- concepts
- how to perform a test in various settings
Week 4: Confidence intervals (CI)
- motivation
- how to construct a CI in various settings
Week 5: Linear regression
- simple and multiple linear regression
- categorical variables
- interpreting output
Week 6: Bootstrap and resampling
- parametric and non-parametric approach
- how to deal with non-standard situations
Tags
Related Courses
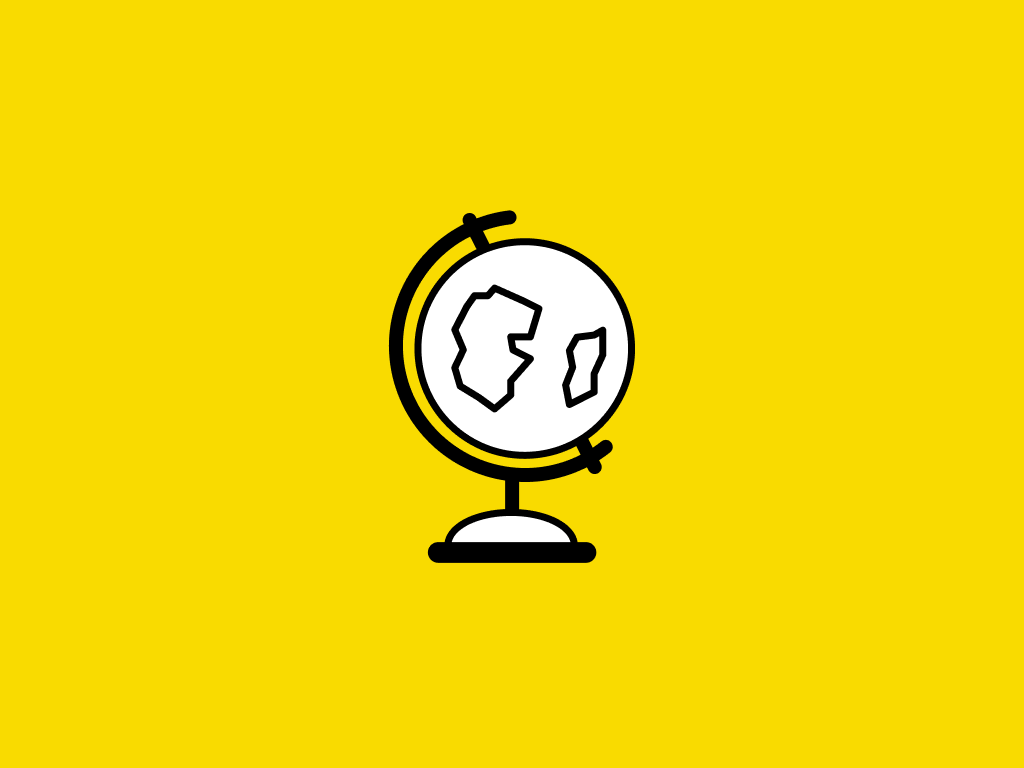
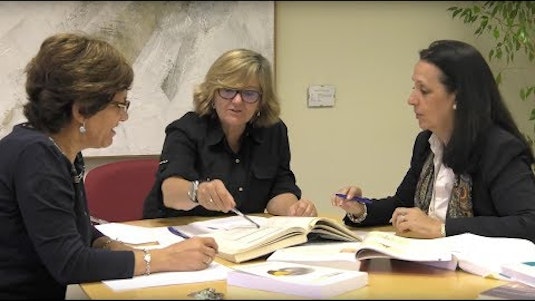
Matemáticas y Estadística: entiéndelas, úsalas
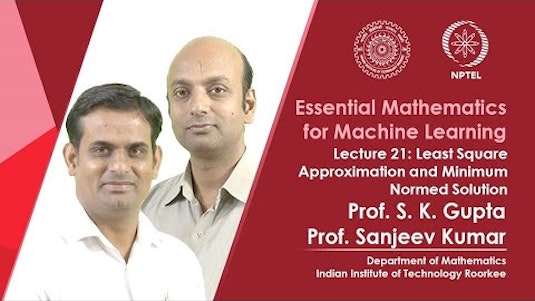
Essential Mathematics For Machine Learning
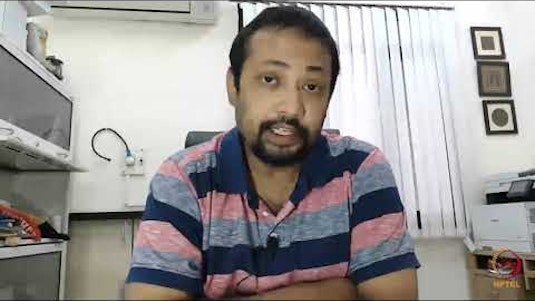
Statistical Mechanics
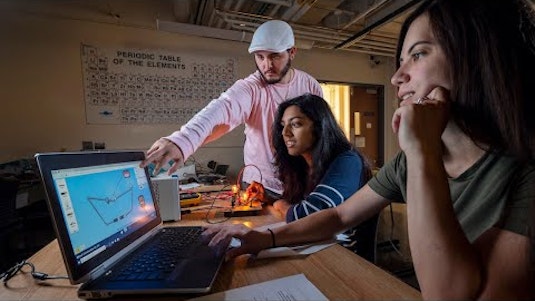
PhETs - Interactive Simulations for Science and maths
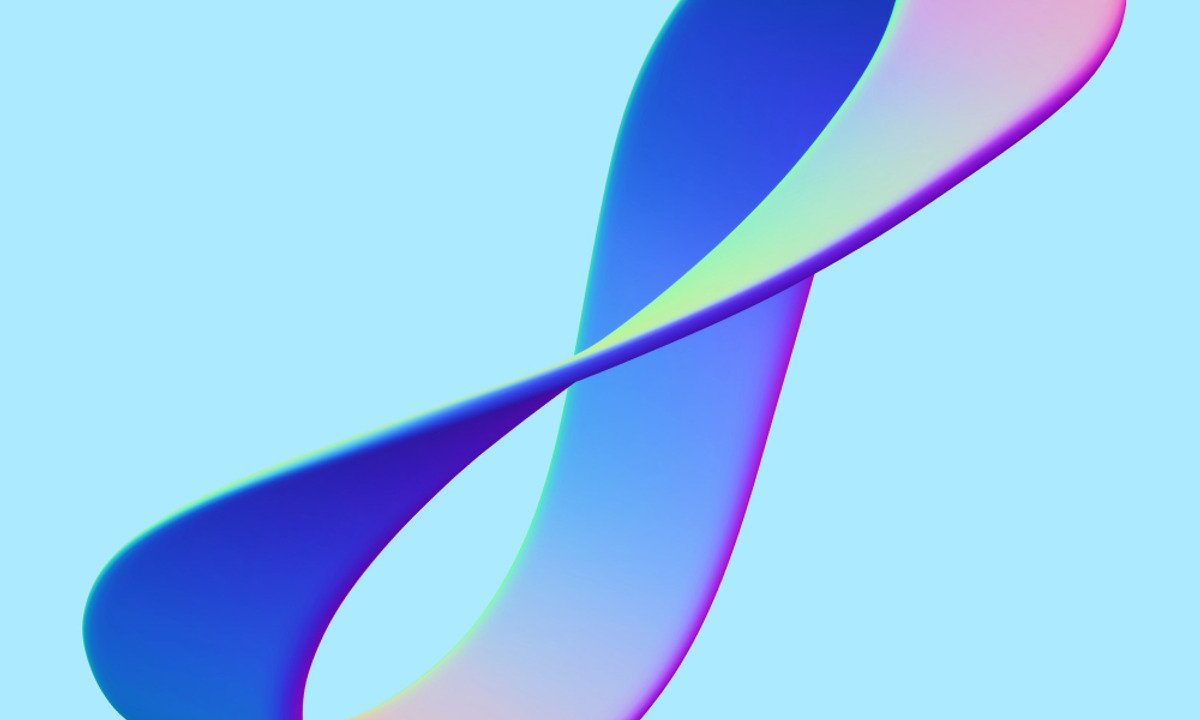
Введение в гладкие многообразия
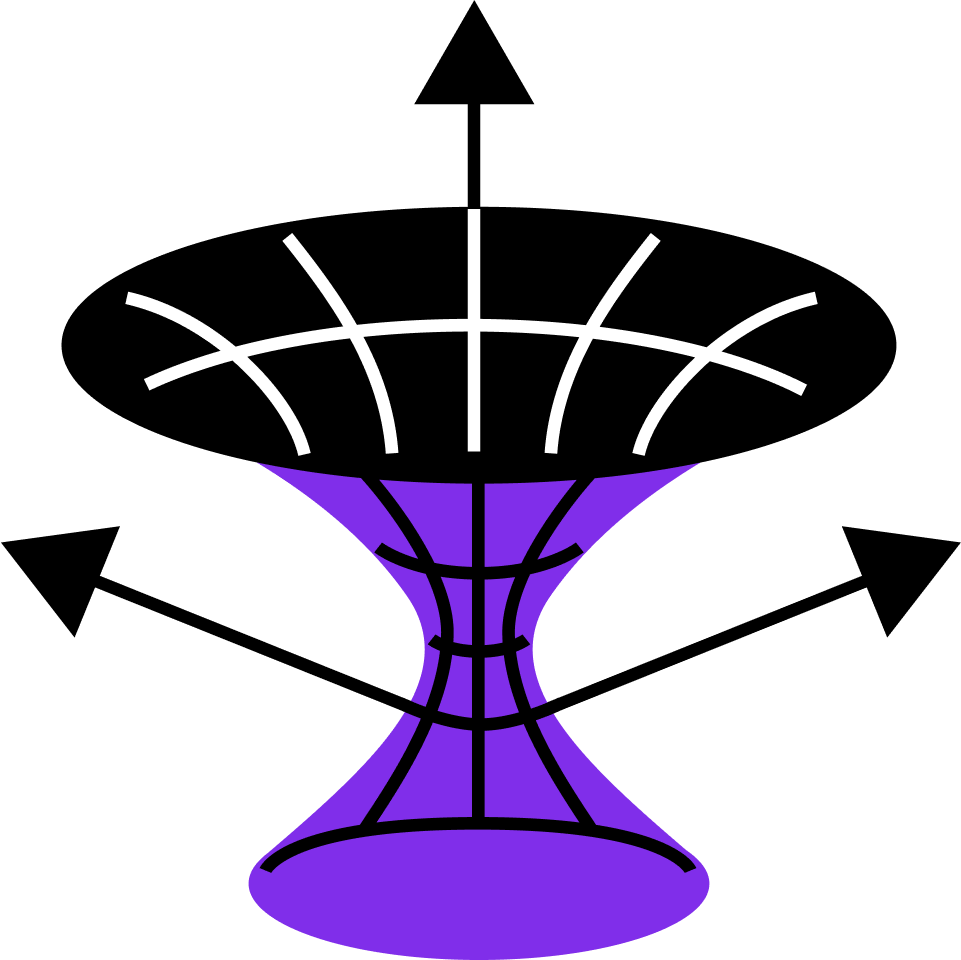
Multivariable Calculus
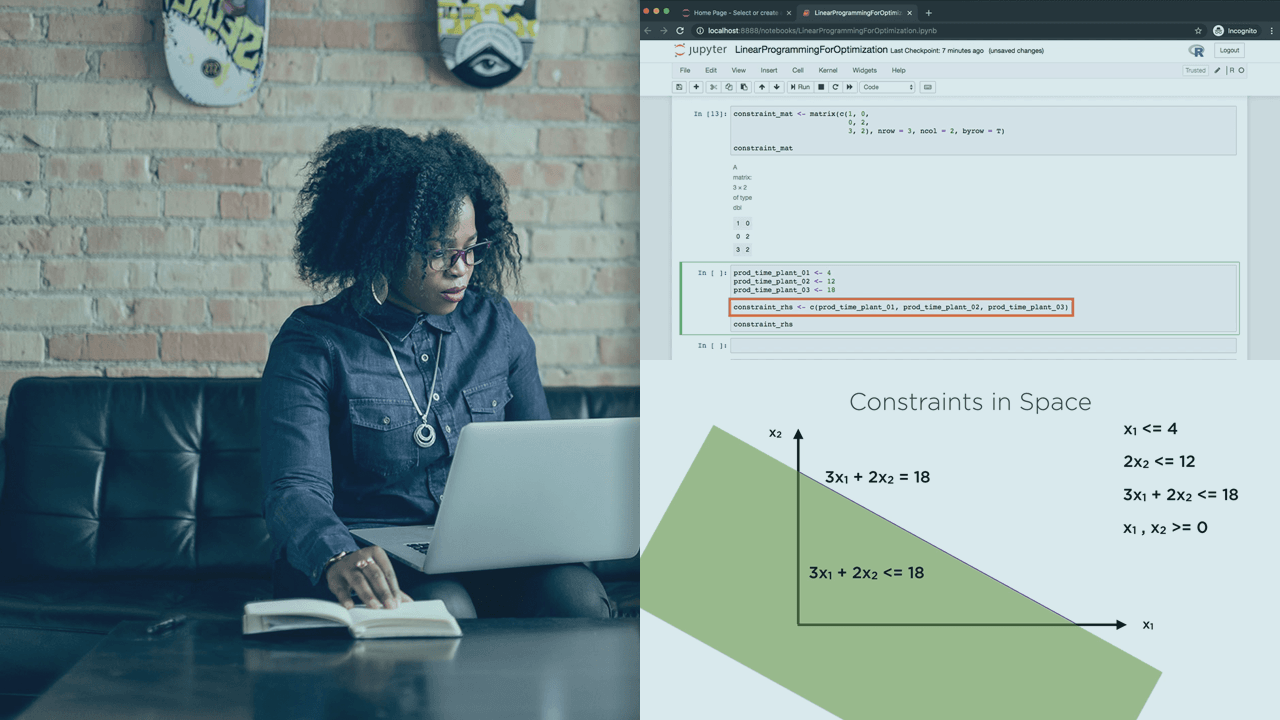
Solving Problems with Numerical Methods
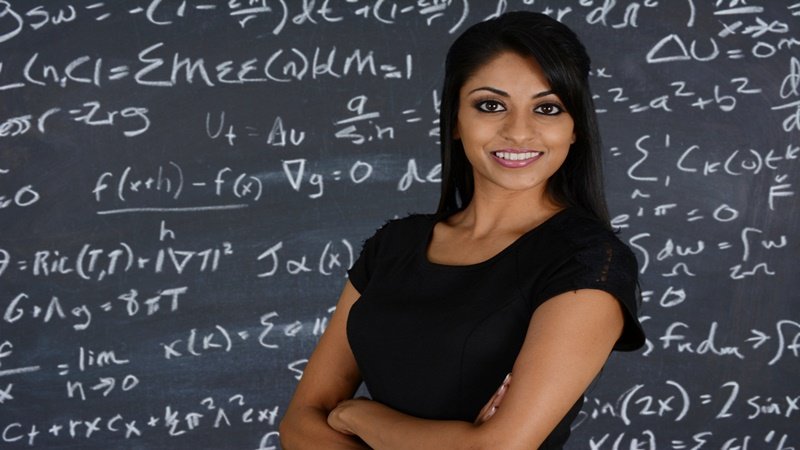
UExcel Precalculus Algebra: Study Guide & Test Prep
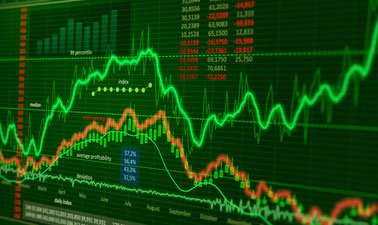
Financial Math for Actuaries: From Loans to Bonds to Immunization
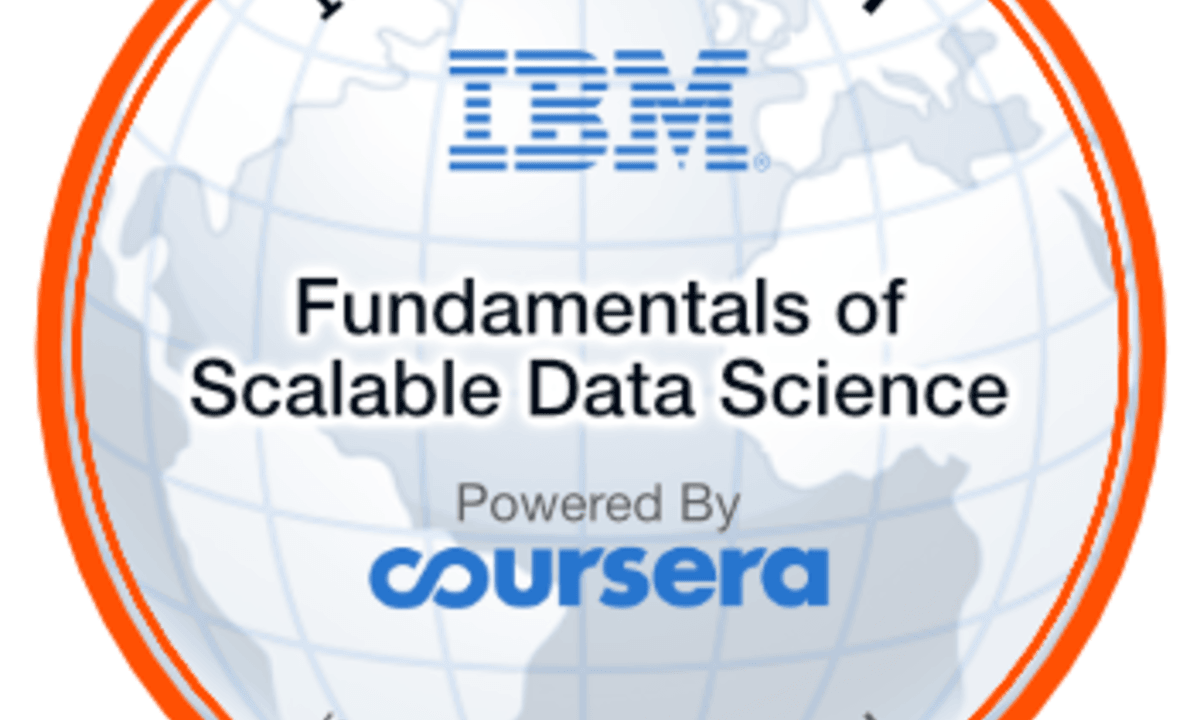
Fundamentals of Scalable Data Science
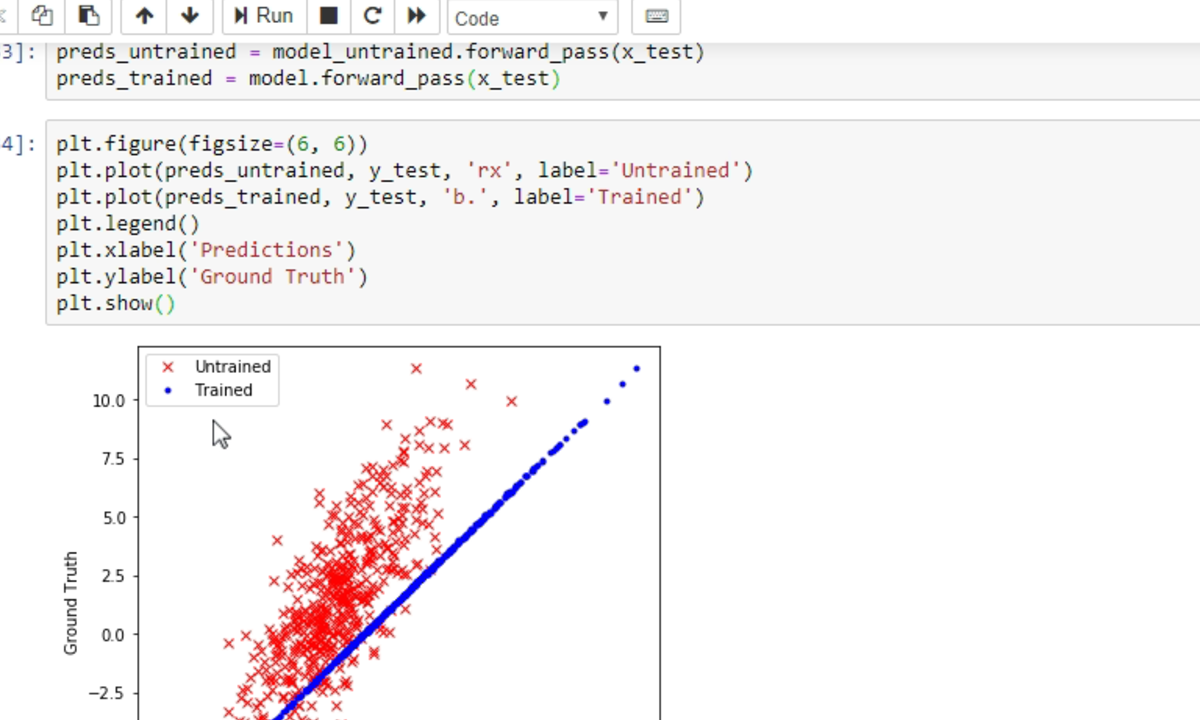