Description
In this course, you will look at models and approaches that are designed to deal with challenges raised by time series data. The discussion covers the motivation for the use of particular models and the description of the characteristics of time series data, with a special attention raised to the potential memory. You will: β Discuss time series models, that refer to data that have been collected over a period on one or more variables for the same individual. β Explore both on stationary and non-stationary time series models, as well as the difference between the non-stationary data and the trend-stationary processes β Consider the problems that may occur with non-stationarity data. β Discover the applications of time series models that are of use when we want to model the GDP growth of an economy, and to test for the Purchasing Power Parity Hypothesis. β Explore the idea of forecasting using econometric models. β Discuss different criteria to decide how good your in-sample and out-of-sample forecasts are. β Explore the problem raised by data where the variance is non-constant, and models for volatility forecasting. β Estimate ARCH(p) and GARCH(p,q) models for volatility with real financial market data and present how to extend these models to the mean of the time series via Garch-in-mean. It is recommended that you have completed and understood the previous three courses in this Specialisation: The Classical Linear Regression Model, Hypothesis Testing in Econometrics and Topics in Applied Econometrics. By the end of this course, you will be able to: β Manipulate and plot the different types of data β Estimate and interpret the empirical autocorrelation function β Estimate and compare models for stationary series β Test for non-stationarity of time series data β Estimate and interpret cointegration equations β Perform in-sample and out-of-sample forecasting exercises β Estimate and compare models for changing volatility
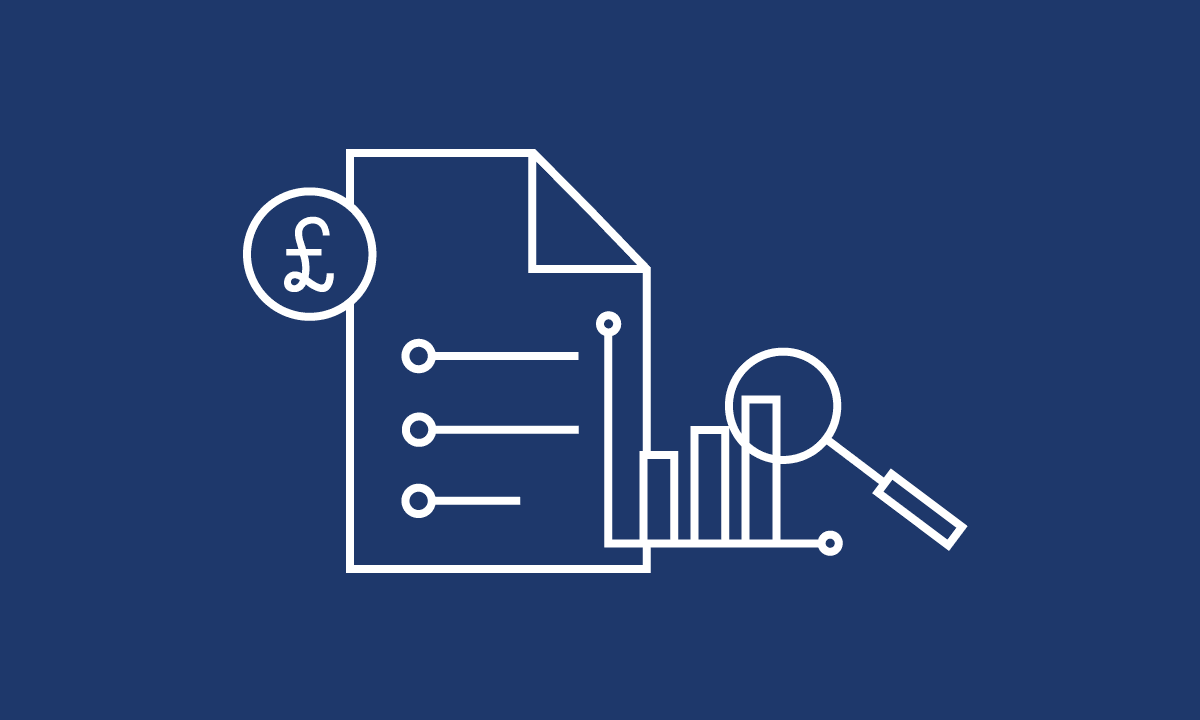
The Econometrics of Time Series Data
-
TypeOnline Courses
-
ProviderCoursera