Description
This statistics and data analysis course will pave the statistical foundation for our discussion on data science.
You will learn how data scientists exercise statistical thinking in designing data collection, derive insights from visualizing data, obtain supporting evidence for data-based decisions and construct models for predicting future trends from data.
Syllabus
Week 1 β Introduction to Data Science
Week 2 β Statistical Thinking
- Examples of Statistical Thinking
- Numerical Data, Summary Statistics
- From Population to Sampled Data
- Different Types of Biases
- Introduction to Probability
- Introduction to Statistical Inference
Week 3 β Statistical Thinking 2
- Association and Dependence
- Association and Causation
- Conditional Probability and Bayes Rule
- Simpsons Paradox, Confounding
- Introduction to Linear Regression
- Special Regression Models
Week 4 β Exploratory Data Analysis and Visualization
- Goals of statistical graphics and data visualization
- Graphs of Data
- Graphs of Fitted Models
- Graphs to Check Fitted Models
- What makes a good graph?
- Principles of graphics
Week 5 β Introduction to Bayesian Modeling
- Bayesian inference: combining models and data in a forecasting problem
- Bayesian hierarchical modeling for studying public opinion
- Bayesian modeling for Big Data
Related Problem Sets
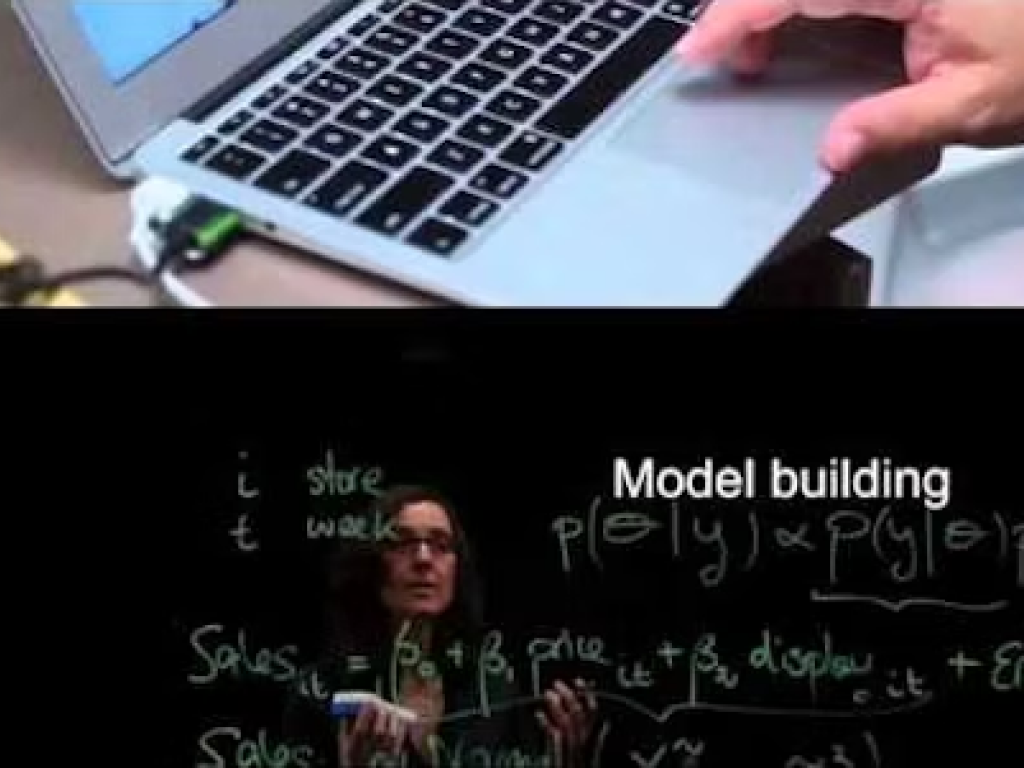
Statistical Thinking for Data Science and Analytics
-
TypeOnline Course
-
Provider
-
PricingFree to Audit
-
CertificatePaid certificate
This statistics and data analysis course will pave the statistical foundation for our discussion on data science.
You will learn how data scientists exercise statistical thinking in designing data collection, derive insights from visualizing data, obtain supporting evidence for data-based decisions and construct models for predicting future trends from data.
Week 1 β Introduction to Data Science
Week 2 β Statistical Thinking
- Examples of Statistical Thinking
- Numerical Data, Summary Statistics
- From Population to Sampled Data
- Different Types of Biases
- Introduction to Probability
- Introduction to Statistical Inference
Week 3 β Statistical Thinking 2
- Association and Dependence
- Association and Causation
- Conditional Probability and Bayes Rule
- Simpsons Paradox, Confounding
- Introduction to Linear Regression
- Special Regression Models
Week 4 β Exploratory Data Analysis and Visualization
- Goals of statistical graphics and data visualization
- Graphs of Data
- Graphs of Fitted Models
- Graphs to Check Fitted Models
- What makes a good graph?
- Principles of graphics
Week 5 β Introduction to Bayesian Modeling
- Bayesian inference: combining models and data in a forecasting problem
- Bayesian hierarchical modeling for studying public opinion
- Bayesian modeling for Big Data